The goal of anpan is to consolidate statistical methods for strain analysis. This includes automated filtering of metagenomic functional profiles, testing genetic elements for association with outcomes, within-species phylogenetic modeling, and abundance-adjusted mixed effects models for microbial pathways.
User manual || Forum || Tutorial
Citation:
TBD (Please feel free to link to anpan in your Methods)
The goal of anpan is to consolidate statistical methods for strain analysis. This includes automated filtering of metagenomic functional profiles, testing genetic elements for association with outcomes, phylogenetic association testing, and pathway-level random effects models.
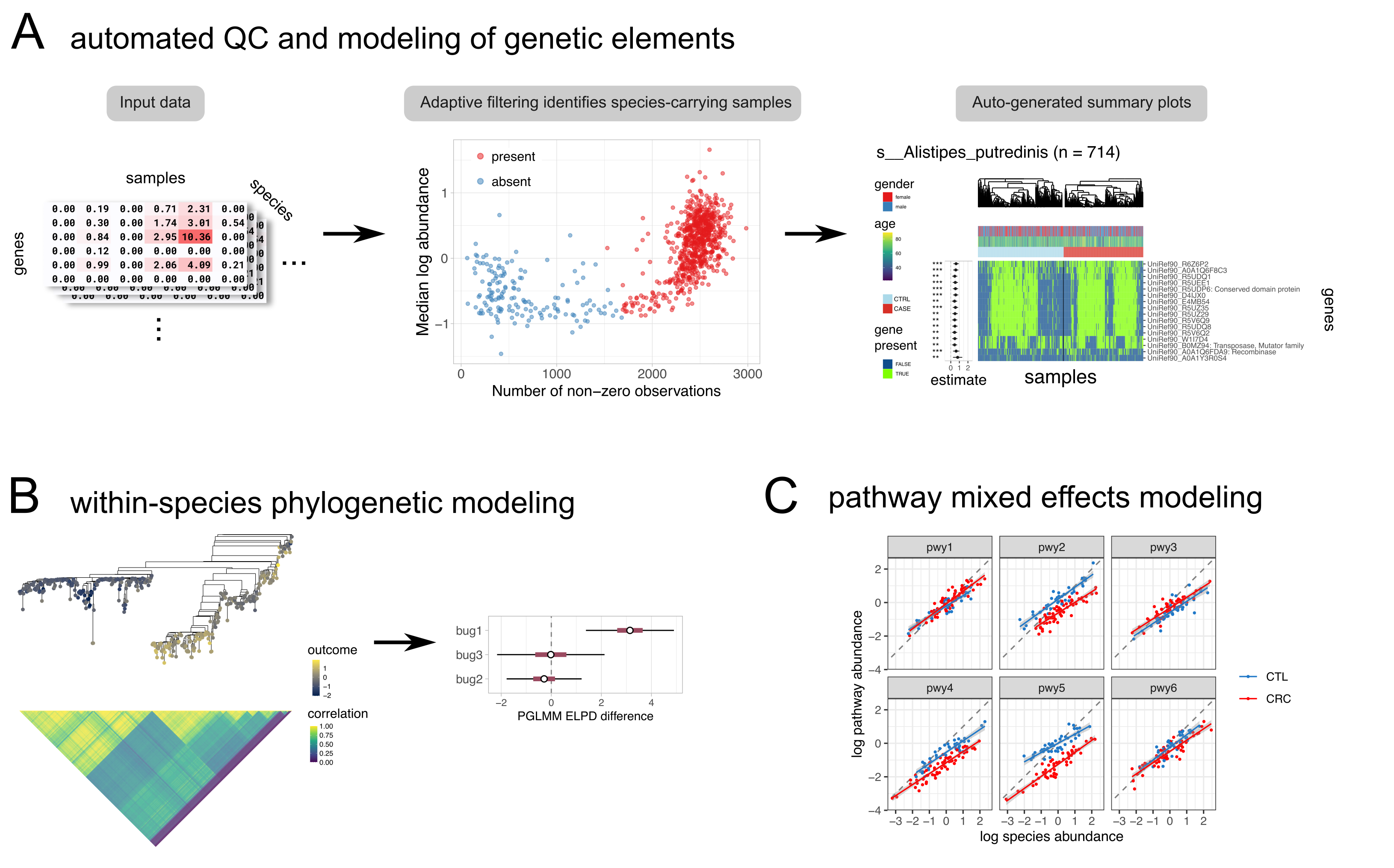
Dependencies
anpan depends on R >= 4.1 and the following R packages, most of which are available through CRAN (the exception being cmdstanr):
install.packages(c("ape",
"data.table",
"dplyr",
"fastglm",
"furrr",
"ggdendro",
"ggnewscale",
"ggplot2",
"loo",
"patchwork",
"phylogram",
"posterior",
"progressr",
"purrr",
"R.utils",
"remotes",
"stringr",
"tibble",
"tidyselect")) # add Ncpus = 4 to go faster
install.packages("cmdstanr", repos = c("https://mc-stan.org/r-packages/", getOption("repos")))
If the cmdstanr
installation doesn’t work you can find more detailed instructions at this link. Once you’ve installed cmdstanr
, you will need to use it to install CmdStan itself:
library(cmdstanr)
check_cmdstan_toolchain()
install_cmdstan(cores = 2)
Installation
Once you have the dependencies, you can install anpan from github with:
remotes::install_github("biobakery/anpan")
If you would like to read the walkthrough vignette, you will need to set the build_vignettes = TRUE
argument in theinstall_github()
command, at which point you can view the vignette with vignette("anpan_tutorial", package = 'anpan')
. There is also a static (and probably not perfectly up-to-date) version of the tutorial available on the biobakery wiki GitHub page.
Example - element testing
You can filter large uniref tables and look for associations with outcomes (while controlling for covariates) withanpan_batch
.
library(anpan)
anpan_batch(bug_dir = "/path/to/gene_families/",
meta_file = "/path/to/metadata.tsv",
out_dir = "/path/to/output",
annotation_file = "/path/to/annotation.tsv", #optional, used for plots
filtering_method = "kmeans",
model_type = "fastglm",
covariates = c("age", "gender"),
outcome = "crc",
plot_ext = "pdf",
save_filter_stats = TRUE)
This will run an anpan batch analysis for each functional profile present in bug_dir
(there should be one input file per bug). For each bug, anpan
:
- reads in the metadata (containing the specified outcome and covariates) and the functional profile
- applies adaptive filtering to discard samples where the species was likely not present (based on low abundance and few non-zero observations) based on the sample’s functional profile.
- fits a model to the filtered data:
model_type = "fastglm"
ormodel_type = "glm"
will fit regressions to each gene in the input file separately (using the specified outcome distribution), then apply FDR correction to the p-values associated with each gene’s presence. The regression for each individual gene follows the form:outcome ~ covariate1 + covariate2 + ... + gene_presence
model_type = "horseshoe"
fits a single regression model with the specified outcome distribution, weak priors on the specified covariates, and a regularized horseshoe prior on the presence/absence of all genes associated with the bug. The regression follows the form:outcome ~ covariate1 + covariate2 + ... + gene1_presence + gene2_presence + gene3_presence + ...
including all of the specified covariates and (typically thousands of) genes detected in the bug.
- then writes results to the output directory, including tables of regression coefficients, per-bug plots of the top hits, and filter statistics.
Example - phylogenetic generalized linear mixed model
You can run a PGLMM with the anpan_pglmm
function. This fits a model where the covariance matrix of outcomes is determined from the phylogenetic tree. Right now the only usable outcome distributions are family = "gaussian"
and family = "binomial"
. If family = "gaussian"
, the reg_noise
parameter can be used to regularize the ratio of the noise parameters with the following prior distribution:
meta_file = "/path/to/metadata.tsv" tree_file = "/path/to/file.tre" anpan_pglmm(meta_file, tree_file, trim_pattern = "_bowtie2", covariates = NULL, plot_cov_mat = TRUE, outcome = "age", verbose = TRUE, cores = 4)
There is a corresponding anpan_pglmm_batch() function that can be pointed to a directory of .tree files with the tree_dir parameter.