About Us
It might seem hard to get excited about a phrase like “microbial communities,” but there’s a whole world of invisible, unknown biology literally almost anywhere you look - much of it with the potential to revolutionize human health. The human body, almost every surface around us, every leaf, and every drop of water contains trillions of microbes - often bacteria, but also viruses, fungi, archaea, and a handful of other microscopic single-celled and multicellular organisms. Microbes were the world’s first chemists, and everything from antibiotics to your ability to digest food and synthesize certain vitamins is thanks to a billion years of microbial evolution before the first eukaryotes emerged. This results in a breathtaking array of inter-microbial warfare, molecular signaling, clever cellular architecture, and enzymatic activity - and that’s before adding in the arms race that pits your immune system against microbial friends and foes alike every day.
The past decade of biotechnology development has allowed us to see this microbial universe in better detail than ever before, using methods like high-throughput DNA sequencing. These and other types of molecular profiling can skip the need to grow every bug of interest individually in the lab. If anything, this has turned a vast pool of unknown-unknowns into a surprising number of known-but-still-unknowns: over two-thirds of the genes in most microbial communities are uncharacterized, often 90% or more, and in the human microbiome alone this leaves millions of microbial proteins interacting in ways that we still don’t understand.
Microbial communities associated with the human body - the human microbiome - are thus of particular interest as a way to better diagnose and treat disease, and as a way to maintain health and wellness. The human microbiome resides primarily in the gut, but communities throughout our body - in the mouth, on the skin, in our nostrils and lungs - influence everything from wound healing to cancer risk. The gut microbiome is particularly interesting as the site of greatest interaction between resident microbes and our immune system, and it most directly influences disease both in the gastrointestinal tract and throughout the body. The gut microbiome can be used to prospectively diagnose colorectal cancer, to predict responses to cancer therapies, to change how we extract nutrients from food or respond to drug treatments, and to fight off systemic diseases like arthritis or diabetes. I personally find microbial communities to be an amazing scientific challenge, and their application to precision medicine in the human microbiome to be one of the greatest opportunities in modern life sciences.
Research Areas
In order to tackle these challenges, the lab works on a variety of areas related to understanding microbial community function and applying it to improve human health:
Major Projects and Resources

The Harvard Chan Center for the Microbiome in Public Health (HCMPH), with the mission of expanding our understanding of the microbiome to improve population health through basic research, translation, policy, education, and outreach. This includes human microbiome contributions to and interactions with chronic disease, basic and infectious disease microbiology, molecular epidemiology, nutrition, environmental health, computational and quantitative methods, and public health policy and best practices.
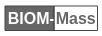
The HCMPH includes the BIOM-Mass platform for microbiome population studies, which provides end-to-end capabilities for microbiome sample collection, handling, data generation, and analysis. These are facilitated by the Harvard Chan Microbiome Collection Core and the Harvard Chan Microbiome Analysis Core.

The lab’s bioBakery software suite is a free, open-source platform for microbial community analysis, focusing on shotgun meta’omic and integrative multi’omic community profiling. It includes facilities for raw microbial community data handling and quality control, taxonomic and functional analysis, and downstream human and environmental population statistics.

The first phase of the Human Microbiome Project (HMP1) characterized the microbial communities from 300 healthy individuals across several different sites on the human body: nasal passages, oral cavity, skin, gastrointestinal tract, and urogenital tract, resulting in a total of over 5,600 16S rRNA gene amplicon profiles and >2,400 shotgun metagenomes from up to three time points per subject.

Our portion of the second phase of the Integrative Human Microbiome Project (HMP2 or iHMP) resulted in the Inflammatory Bowel Disease Multi’omics Database (IBDMDB), which followed 132 subjects from five clinical centers over the course of one year each. Integrated longitudinal molecular profiles for over 1,700 biweekly stool samples, >650 intestinal biopsies, and >500 quarterly blood draws (metagenomes, metatranscriptomes, metaproteomes, viromes, metabolomes, host exomes, epigenomes, transcriptomes, and serological profiles) are available at the IBDMDB data portal, along with protocols and further resources from the study.
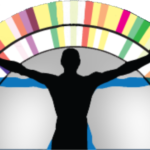
The Microbiome Quality Control Project (MBQC) performed a first evaluation of two of the several steps typically used to obtain and analyze the human microbiome. The baseline assessment included contributions from 16 sample handling laboratories and nine bioinformatics laboratories, in addition to several additional groups participating in data analysis and manuscript preparation - all on a much-appreciated volunteer basis! The resulting baseline data include raw sequences, sequence data re-blinded prior to bioinformatics processing, raw OTU tables, and the final integrated data products.
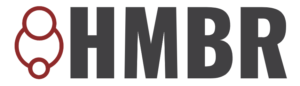
The Human Microbiome Bioactives Resource (HMBR) provides a comprehensive platform for discovery, validation, and early-stage translation of novel therapeutics derived from the microbiome, including protocols for microbiome multi’omic sampling and profiling, meta-analysis methods and results for tens of thousands of standardized gut microbiome profiles, prioritized potentially bioactive elements of the gut microbiome in IBD (microbial species and strains, proteins, secreted peptides, biosynthetic gene clusters, and small molecules), and screens for high-priority bioactives in mammalian cell and tissue culture and in animal models.